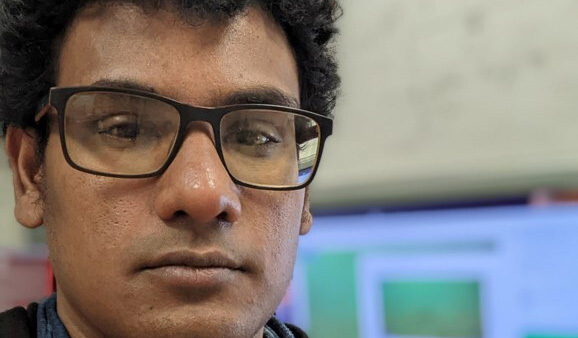
“Meet Our Grad Student Scholars” is an article series from Illinois-Indiana Sea Grant (IISG) celebrating the graduate students doing research funded by the IISG scholars program. To learn more about our faculty and graduate student funding opportunities, visit our Fellowships & Scholarships page.
Arunim Bhattacharya is a doctoral student in the Emergent & Autonomous Systems Laboratory at Northern Illinois University and part of the inaugural Illinois-Indiana Sea Grant graduate student scholars cohort. He is interested in invasive species monitoring in the Great Lakes region and currently seeks to identify aquatic invasive species (AIS) using machine learning techniques. He is also interested in studying interactions between human AIS monitoring and robot-swarm AIS monitoring. Robot swarms can explore a large environment, but humans have better situational awareness, and Arunim is particularly interested in how these strengths can complement one another.
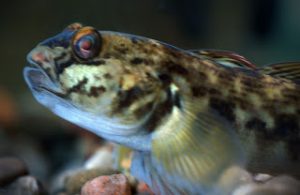
Closeup of a round goby. Photo credit Michigan Sea Grant.
Aquatic invasive species remain a major threat to Great Lakes ecosystems, and Arunim Bhattacharya, a PhD student at Northern Illinois University, is using machine learning techniques to help. Round gobies, a fish introduced to the Great Lakes in the 1990s, have since invaded many nearshore regions. Scientists know they are playing key roles in local ecosystems, but the round goby’s tendency to live in rocky bottom areas, plus their cryptic coloring, makes them very hard to detect.
One method that scientists have used is to take pictures or video of the lake bottom, then count the round gobies that are present. In some cases, humans do the counting, while in others, computers do it. Unfortunately, different species of fish can look similar on camera, especially in murky waters with a cluttered background. Through his research, Arunim is developing a clever way around this issue: instead of identifying round goby by the way they look, Arunim has found a way to identify them by the way they move. He is using machine learning techniques to identify round goby movement in videos, which will allow scientists to better understand the role they are playing in Great Lakes ecosystems and (hopefully) slow or stop expansions to new areas.
To teach a computer to identify and recognize round goby, Arunim is creating a virtual aquatic world. His model Great Lake includes five fish species: common carp, lake perch, lake whitefish, yellow perch, and round goby. The virtual aquarium is based on videos of fish that had been gathered on the citizen (or community) science platform Zooniverse as part of the Deep Lake Explorer project. Frame-by-frame, Arunim identifies the round goby and annotates the video using a software called Peregrine. In addition to drawing a box around the fish, Arunim tries to identify specific behaviors, like foraging, shoaling, which is swimming together, and any quick jittery movements to and from the school.
“The idea is if I have one or two minutes of footage, I see what percentage of that is fish schooling or shoaling and that gives me an idea of how to code them to behave in the virtual reality setup,” said Arunim. Though the videos are relatively short, the process of annotating each one takes 2-4 hours. It is important, though, as this training of the machine algorithm sets up the next part of Arunim’s work: training a computer.
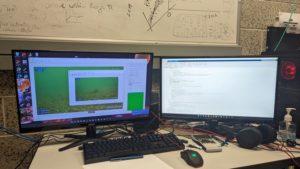
Arunim uses two computer screens while annotating videos.
Like all machine learning projects, this algorithm needs to be developed using an immense amount of data to increase its ultimate accuracy. That’s one of the reasons Arunim likes the Zooniverse-sourced videos: they provide lots of data without being intrusive or costly.
The annotated video footage is then fed through a machine learning algorithm—machine learning refers to how computers can generalize some behavior, for example how a particular fish species moves without a human needing to program that behavior. The algorithm trains on trajectory data: the squares Arunim drew around the fish in the first part of his research and the percentages of types of movement he identified. Arunim uses equations to help understand the optimal, or “just right,” model that explain the information well, but do not try to add a lot of extra information. Models with more extra information are “overfit” and may explain current conditions well, but typically do not perform as well when used to explore new videos or information.
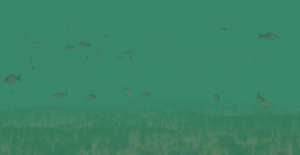
An example of a simulated underwater aquatic world that Arunim will create.
Once the learning algorithm has trained on enough data, it will be able to identify round goby in new, unannotated footage. When an unknown trajectory is passed through the program, it will output the probability that the trajectory belongs to round goby.
The type of program Arunim uses here is called a neural network, because it behaves a bit like a human brain with respect to how it pulls data together, groups it, analyzes it, and sends it out again. By tracking organisms and matching their trajectories, Arunim’s program will be able to ultimately identify round goby in brand new videos captured from the wild.
This machine learning is part of a bigger project—making underwater robots that will be able to capture and identify footage to mitigate round goby and their ecological damage If it is successful, the algorithm could be used to identify other species.
Arunim’s advice to students considering graduate school is to find a great advisor who will be supportive, and just go for it.
You can learn more about Arunim’s research by following @ArunimBhattach1 on Twitter.
Illinois-Indiana Sea Grant is a part of University of Illinois Extension and Purdue Extension.
Writer: Sarah Gediman
Contact: Carolyn Foley